- April 19, 2024
- Posted by: GMAS Team
- Category: Blog
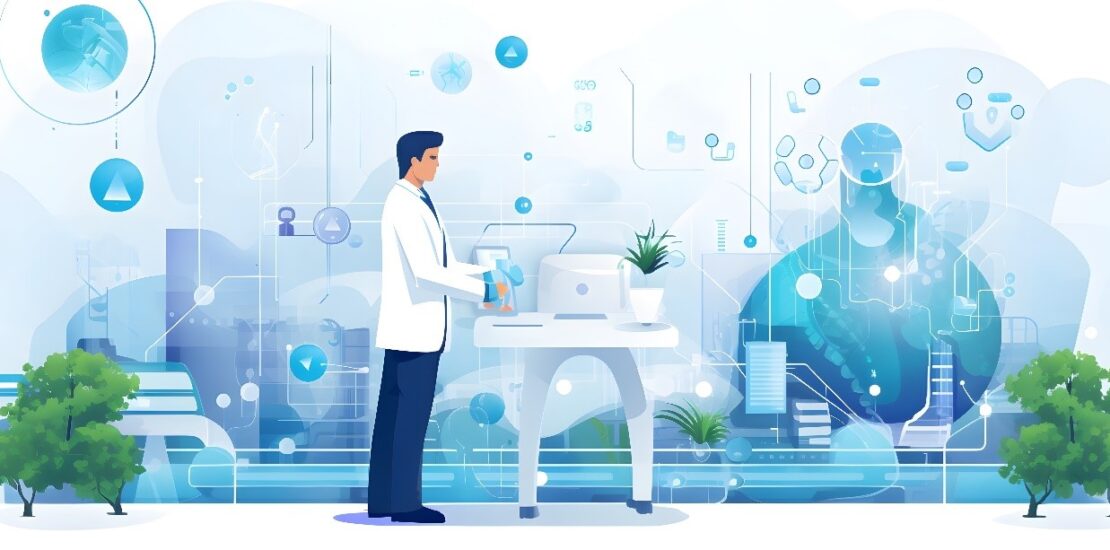
The healthcare sector is on the verge of a groundbreaking period, propelled by the merging of advanced technologies and insights derived from data analysis. Among these innovations, predictive analytics emerges as a powerful tool, reshaping how healthcare providers deliver care, manage resources, and improve patient outcomes. By leveraging predictive models and advanced algorithms, healthcare organizations can anticipate trends, identify risk factors, and tailor interventions to individual patient needs. In this blog, we delve into the multifaceted applications of predictive analytics in healthcare, highlighting its profound impact on early disease detection, personalized treatment, resource optimization, medication adherence, and population health management.
Early Disease Detection and Prevention:
Early detection of diseases is pivotal in mitigating their impact and improving patient outcomes. Predictive analytics empowers healthcare providers to identify individuals at risk of developing various health conditions, ranging from chronic diseases like diabetes and cardiovascular disorders to infectious diseases such as COVID-19. By analyzing a plethora of data sources, including electronic health records, genetic profiles, lifestyle factors, and environmental variables, predictive models can stratify populations based on their susceptibility to specific diseases. For instance, in diabetic care, it can flag patients with elevated risk scores, prompting timely interventions such as lifestyle modifications, preventive screenings, or pharmacological treatments to prevent disease progression and complications.
Personalized Treatment Plans:
Healthcare is increasingly moving towards a patient-centric approach, emphasizing personalized treatments tailored to individual characteristics and preferences. Predictive analytics plays a pivotal role in this paradigm shift by harnessing patient data to optimize treatment decisions and improve therapeutic outcomes. By integrating genomic information, clinical data, and real-time monitoring metrics, models can predict patient responses to different treatment modalities, guiding clinicians in selecting the most effective and least invasive interventions. For instance, in cancer care, it can help oncologists identify genetic biomarkers associated with treatment response, enabling the customization of chemotherapy regimens to maximize efficacy and minimize adverse effects, thus improving patient survival rates and quality of life.
Hospital Resource Optimization:
Efficient allocation of resources is paramount for healthcare institutions to meet patient demands while ensuring operational sustainability. Predictive analytics offers invaluable insights into resource utilization patterns, enabling hospitals to optimize bed capacity, staffing levels, and supply chain management. By analyzing historical data, patient flow dynamics, and demographic trends, predictive models can forecast patient admissions, emergency department utilization, and critical care needs with remarkable accuracy. For example, it can predict seasonal fluctuations in patient volumes, enabling hospitals to adjust staffing levels accordingly and preemptively allocate resources to areas with the highest demand, thus reducing wait times, enhancing patient satisfaction, and optimizing operational efficiency.
Improved Medication Adherence:
Non-adherence to medication regimens poses a significant challenge in managing chronic diseases and preventing disease exacerbations. Predictive analytics offers a proactive solution by identifying patients at risk of non-adherence and implementing targeted interventions to promote medication compliance. By analyzing prescription patterns, patient demographics, socio-economic factors, and behavioral indicators, predictive models can identify individuals predisposed to medication non-compliance. Healthcare providers can then intervene through personalized interventions such as medication reminders, patient education programs, or telehealth consultations to support patients in adhering to their treatment plans, thereby reducing hospital readmissions, preventing disease complications, and improving overall health outcomes.
Enhanced Population Health Management:
Population health management endeavors to improve the health outcomes of entire communities by addressing prevalent health risks, promoting preventive care, and optimizing healthcare delivery. Predictive analytics serves as a cornerstone of population health management by identifying high-risk populations, predicting disease outbreaks, and targeting interventions to address underlying health disparities. By aggregating and analyzing data from diverse sources such as electronic health records, public health databases, and social determinants of health, predictive models can identify population segments at elevated risk of specific health conditions. Healthcare organizations can then deploy proactive interventions such as community outreach programs, vaccination campaigns, and health education initiatives to address these disparities and improve the overall health and well-being of the population.
Optimized Revenue Cycle Management (RCM):
Predictive analytics is not just transforming clinical care; it’s revolutionizing revenue cycle management in healthcare. By analyzing historical billing data, predictive models can anticipate trends in claims denials, payment delays, and coding errors. This foresight enables proactive measures to prevent denials, optimize pricing strategies, and streamline billing processes, ultimately enhancing revenue capture and financial performance.
Efficient Claims Processing:
Predictive analytics streamlines the claims processing workflow by identifying inefficiencies and suggesting improvements. By analyzing patterns in claim submissions and processing, predictive models help healthcare organizations identify bottlenecks and implement process improvements to accelerate revenue collection and reduce administrative burdens.
Fraud Prevention:
In addition to optimizing revenue capture, predictive analytics helps healthcare organizations combat fraud. By analyzing billing patterns and comparing them to historical data, predictive models flag suspicious claims for further investigation, minimizing revenue leakage due to fraudulent activities and ensuring compliance with regulatory requirements.
Predictive analytics holds immense promise in revolutionizing healthcare delivery by empowering providers with actionable insights to enhance early disease detection, personalize treatments, optimize resource allocation, promote medication adherence, and improve population health outcomes. As healthcare continues to evolve in an era of digital transformation, it will undoubtedly remain a cornerstone of innovation, driving advancements in clinical decision-making, patient engagement, and healthcare delivery models. Healthcare organizations can usher in a new era of precision medicine, preventive care, and population health management, ultimately achieving better health results, cutting down on healthcare expenses, and fostering a healthier community overall.