- March 20, 2024
- Posted by: GMAS Team
- Category: Blog
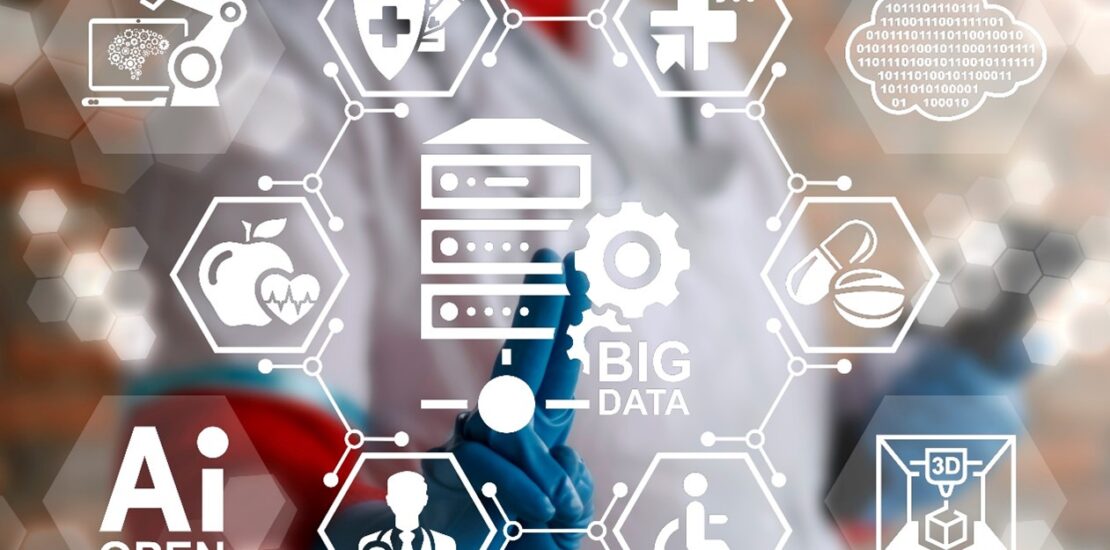
In the ever-evolving landscape of healthcare, the integration of big data analytics has become a transformative force, promising unprecedented insights and improved patient outcomes. As technology continues to advance, so does the potential for leveraging data to enhance healthcare delivery. In this blog, we will delve into the emerging strategies that are shaping the future of big data analytics in healthcare.
The Intersection of Healthcare and Big Data:
Healthcare, traditionally a field driven by expertise and experience, is undergoing a paradigm shift with the infusion of big data analytics. The vast amounts of data generated within the healthcare ecosystem, from electronic health records (EHRs) and medical imaging to wearable devices and genomic information, have laid the foundation for innovative approaches to diagnosis, treatment, and patient care.
Artificial Intelligence and Machine Learning in Diagnostics:
One of the most promising developments in healthcare analytics is the incorporation of artificial intelligence (AI) and machine learning (ML) algorithms into diagnostics. These technologies can analyze vast datasets to identify patterns and anomalies, aiding in the early detection of diseases. From image recognition in radiology to predictive analytics for chronic conditions, AI and ML are revolutionizing the diagnostic process, enabling quicker and more accurate assessments.
As AI algorithms continuously learn and adapt from new data inputs, they become increasingly adept at recognizing subtle nuances that may escape the human eye. This not only accelerates the diagnostic process but also enhances the precision of medical diagnoses, leading to more effective treatment strategies.
Predictive Analytics for Personalized Medicine:
The concept of personalized medicine is gaining traction, and big data analytics plays a pivotal role in making it a reality. By analyzing patient-specific data, including genetic information, lifestyle factors, and treatment histories, predictive analytics can identify optimal treatment plans tailored to individual needs. This not only enhances the efficacy of treatments but also minimizes adverse effects, paving the way for a more patient-centric approach to healthcare.
Predictive analytics algorithms assess a multitude of variables to predict how a patient is likely to respond to different treatment options. This information empowers healthcare providers to make informed decisions, ensuring that interventions are not only effective but also aligned with the unique characteristics of each patient.
Real-Time Data Processing for Enhanced Patient Monitoring:
The ability to monitor patients in real time is a game-changer in healthcare analytics. With the integration of advanced sensors and wearable devices, healthcare providers can collect and analyze continuous streams of data, allowing for early detection of deteriorating health conditions. This real-time data processing enables timely interventions, reducing hospital readmissions and improving overall patient care.
Real-time monitoring provides healthcare professionals with a comprehensive view of a patient’s health status, enabling proactive measures to prevent potential complications. This approach not only improves patient outcomes but also reduces the strain on healthcare resources by minimizing the need for emergency interventions.
Blockchain for Secure and Interoperable Health Data Exchange:
Data security and interoperability have been persistent challenges in healthcare analytics. Blockchain technology offers a decentralized and secure platform for storing and sharing patient data. This not only ensures the integrity and confidentiality of health records but also facilitates seamless data exchange between different healthcare entities. Blockchain’s tamper-resistant nature enhances trust in the accuracy of health information, fostering collaboration among healthcare stakeholders.
Blockchain’s decentralized architecture eliminates the need for a central authority, reducing the risk of data breaches and unauthorized access. By creating a secure and transparent system for data exchange, blockchain promotes interoperability, allowing healthcare providers to access critical information seamlessly while maintaining data integrity.
Integration of Social Determinants of Health (SDOH):
Understanding the social determinants of health is crucial for a comprehensive analysis of patient well-being. Big data analytics now incorporates SDOH data, including factors like socio-economic status, education, and community environment. By considering these broader influences, healthcare providers can develop targeted interventions to address underlying social issues, ultimately improving health outcomes and reducing disparities.
The integration of SDOH data provides a holistic view of a patient’s health, allowing healthcare professionals to address not only the symptoms of a condition but also its root causes. This approach is particularly valuable in population health management, where addressing social determinants can lead to more effective preventive measures and community-based interventions.
Edge Computing for Faster Processing and Reduced Latency:
As the volume of healthcare data continues to grow, the need for faster processing and reduced latency becomes paramount. Edge computing, a distributed computing paradigm, brings data processing closer to the source, reducing the time it takes to analyze and act upon information. In healthcare, this translates to quicker decision-making, particularly in critical situations such as emergency response and surgical procedures.
Edge computing minimizes the reliance on centralized data processing centers, enabling real-time analysis and decision-making at the point of care. This not only enhances the efficiency of healthcare delivery but also supports the implementation of emerging technologies such as telemedicine and remote patient monitoring.
Federated Learning for Privacy-Preserving Analytics:
Patient privacy is a top concern in healthcare analytics. Federated learning addresses this issue by allowing machine learning models to be trained across multiple decentralized devices without sharing raw data. This approach enables healthcare organizations to collaborate on model development while keeping sensitive patient information localized. Privacy-preserving analytics foster trust and compliance with data protection regulations.
Federated learning ensures that patient data remains secure and private, as the raw information never leaves the local device. This collaborative approach to model training promotes knowledge sharing among healthcare institutions without compromising individual privacy, making it an essential strategy for advancing big data analytics in healthcare.
The landscape of big data analytics in healthcare is evolving rapidly, ushering in a new era of personalized and efficient patient care. From the integration of artificial intelligence and machine learning for diagnostics to the use of blockchain for secure data exchange, these emerging strategies are reshaping the way healthcare is delivered and managed. As technology continues to advance, the synergy between data analytics and healthcare holds the promise of not just treating illnesses but predicting and preventing them, ultimately leading to a healthier and more connected world. The future of healthcare is data-driven, and the journey has only just begun.